This is the third in a three-part series introducing revolutionary changes in AI-generated art. In Part I: AI Art Comes of Age, we traced back through some of the winding path that brought us to this point. Part II: Deep Learning and The Diffusion Revolution, 2014-present, introduced three basic methods for generating art via deep-learning networks: GANs, VAEs and Diffusion models.
But what does it all mean? What’s at stake? In this final installment, let’s discuss some of the opportunities, legal and ethical questions presented by these new Engines of Wow.
Opportunities and Disruptions
We suddenly have robots which can turn text prompts into relevant, engaging, surprising images for pennies in a matter of seconds. They can compete with custom-created art taking illustrators and designers days or weeks to create.
Anywhere an image is needed, a robot can now help. We might even see side-by-side image creation with spoken words or written text, in near real-time.
- Videogame designers have an amazing new tool to envision worlds.
- Bloggers, web streamers and video producers can instantly and automatically create background graphics to describe their stories.
- Graphic design firms can quickly make logos or background imagery for presentations, and accelerate their work. Authors can bring their stories to life.
- Architects and storytellers can get inspired by worlds which don’t exist.
- Entire graphic novels can now be generated from a text script which describes the scenes without any human intervention. (The stories themselves can even be created by new Chat models from OpenAI and other players.)
- Storyboards for movies, which once cost hundreds of thousands of dollars to assemble, can soon be generated quickly, just by ingesting a script.
It’s already happening. In the Midjourney chat room, user Marcio84 writes: “I wrote a story 10 years ago, and finally have a tool to represent its characters.” With a few text prompts, the Midjourney Diffusion Engine created these images for him for just a few pennies:
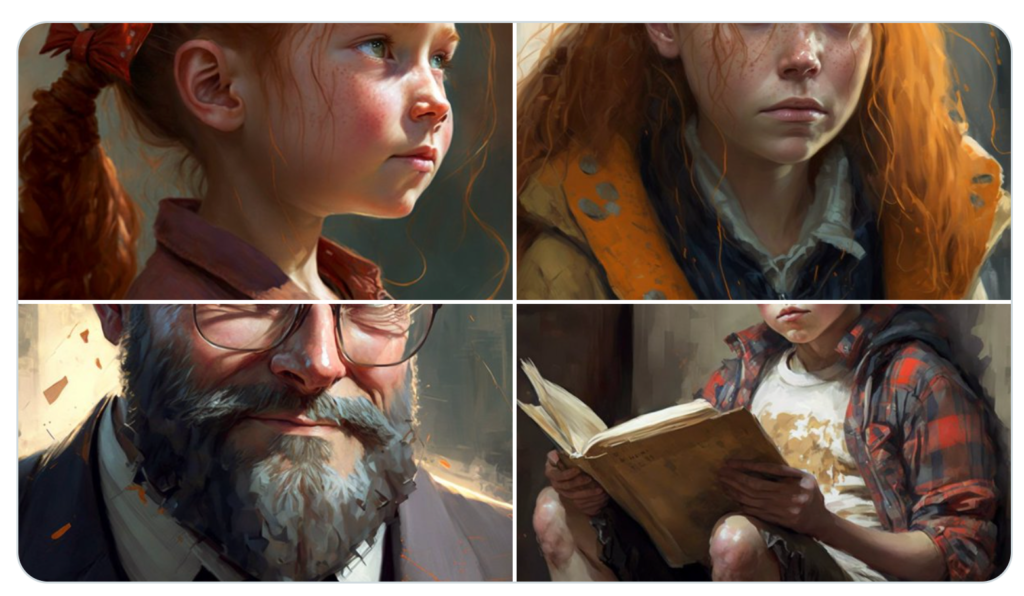
Industrial designers, too, have a magical new tool. Inspiration for new vehicles can appear by the dozens and be voted up or down by a community:
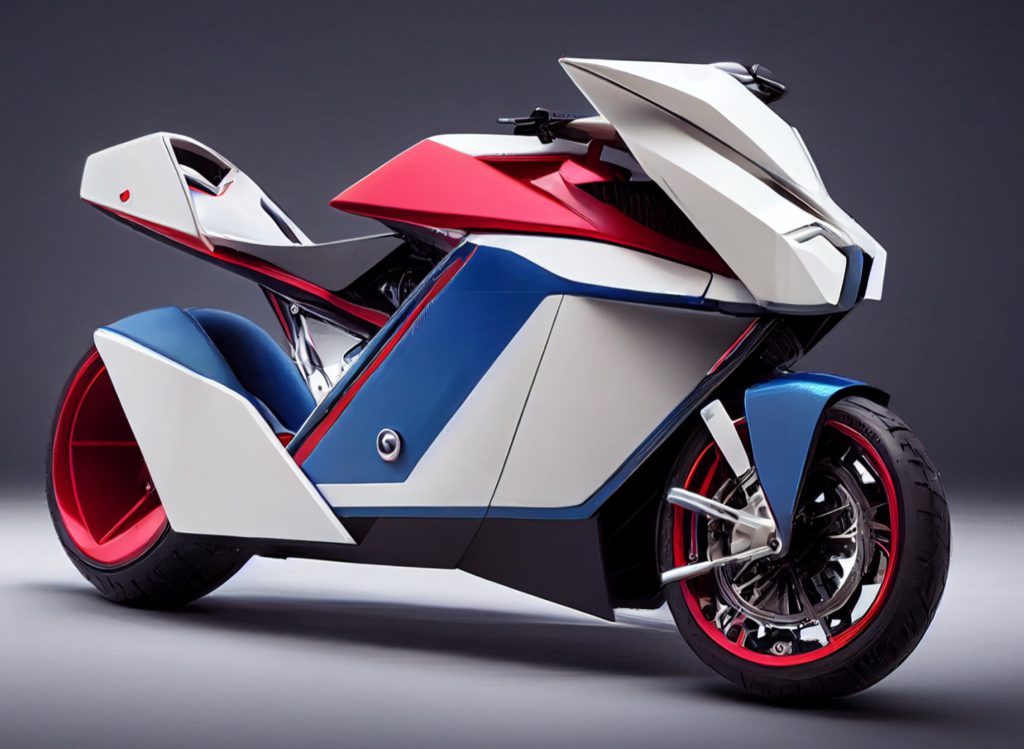
These engines are capable of competing with humans. In some surveys, as many as 87% of respondents incorrectly felt an AI-generated image was that of a real person. Think you can do better? Take the quiz.
I bet you could sell the art below, generated by Midjourney from a “street scene in the city, snow” prompt, in an art gallery or Etsy shop online. If I spotted it framed on a wall somewhere, or on a book cover or movie poster, I’d have no clue it was computer-generated:

A group of images stitched together becomes a video. One Midjourney user has tried to envision the aging destruction of a room, via successive videoframes of ever-more decaying description:
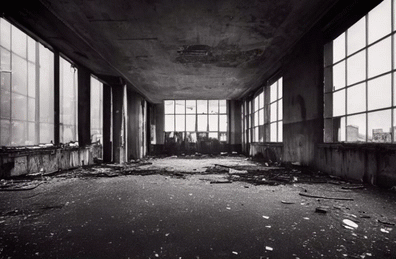
These are just a few of the things we can now do with these new AI art generation tools. Anywhere an image is useful, AI tools will have an impact, by lowering cost, blending concepts and styles, and envisioning many more options.
Where do images have a role? Well, that’s pretty much every field: architecture, graphic design, music, movies, industrial design, journalism, advertising, photography, painting, illustration, logo design, training, software, medicine, marketing, education and more.
Disruption
The first obvious impact is that many millions of employed or employable people may soon have far fewer opportunities.
Looking just at graphic design, approximately half a million designers employed globally, about 265,000 of whom are in the United States. (Source: Matt Moran of Colorlib.) The total market size for graphic design is about $43 billion per year. 90% of graphic designers work freelance, and the Fortune 500 accounts for nearly one-fifth of graphic design employment.
That’s just the graphic design segment. Then, there are photographers, painters, landscape architects and more.
But don’t count out the designers yet. These are merely tools, just as photography ones. And, while the Internet disrupted (or “disintermediated”) brokers in certain markets in the ’90s and ’00s (particularly in travel, in-person retail, financial services and real estate), I don’t expect that AI-generation tools means these experts are obsolete.
But the AI revolution is very likely to reduce the dollars available and reshape what their roles are. For instance, travel agents and financial advisers very much do still exist, though their numbers are far lower. The ones who have survived — even thrived — have used the new tools to imagine new businesses and have moved up the value-creation chain.
Who Owns the Ingested Data? Who Compensates the Artists?
Is this all plagiarism of sorts? There are sure to be lawsuits.
These algorithms rely upon massive image training sets. And there isn’t much dotting of i’s and crossing of t’s to secure digital rights. Recently, an artist found her own private medical records in one publicly available training dataset on the web which has been used by Stability AI. You can check to see if your own images have been part of the training datasets at www.haveibeenstrained.com.
But unlike most plagiarism and “derivative work” lawsuits up until about 2020, these lawsuits will need to contend with not being able to firmly identify just how the works are directly derivative. Current caselaw around derivative works generally requires some degree of sameness or likeness from input to final result. But the body of imagery which go into training the models is vast. A given creative end-product might be associated with hundreds of thousands or even millions of inputs. So how do the original artists get compensated, and how much, if at all?
No matter the algorithm, all generative AI models rely upon enormous datasets, as discussed in Part II. That’s their food. They go nowhere without creative input. And these datasets are the collective work of millions of artists and photographers. While some AI researchers go to great lengths to ensure that the images are copyright-free, many (most?) do not. Web scraping is often used to fetch and assemble images, and then a lot of human effort is put into data-cleansing and labeling.
The sheer scale of “original art” that goes into these engines is vast. It’s not unusual for a model to be trained by 5 million images. So these generative models learn patterns in art from millions of samples, not just by staring at one or two paintings. Are they “clones?” No. Are they even “derivative?” Probably, but not in the same way that George Harrison’s “My Sweet Lord” was derivative of Ronnie Mack’s “He’s So Fine.”
In the art world, American artist Jeff Koons created a collection called Banality, which featured sculptures from pop culture: mostly three dimensional representations of advertisements and kitsch. Fait d’Hiver (Fact of Winter) was one such work, which sold for approximately $4.3 million in a Christie’s auction in 2007:
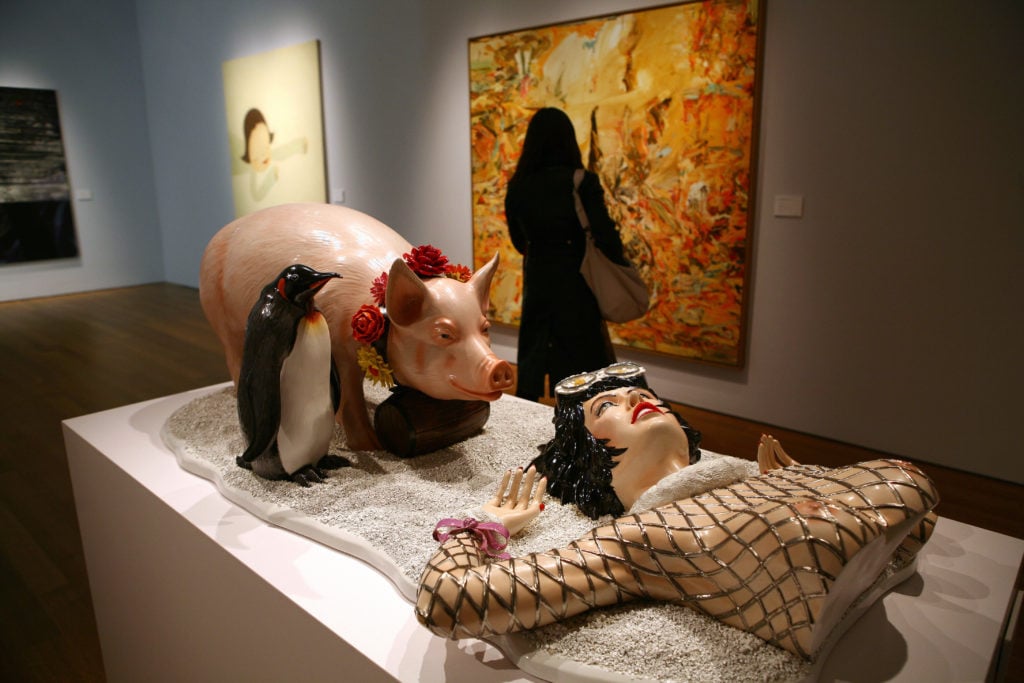
Davidovici acknowledged that his sculpture was both inspired by and derived from this advertisement:
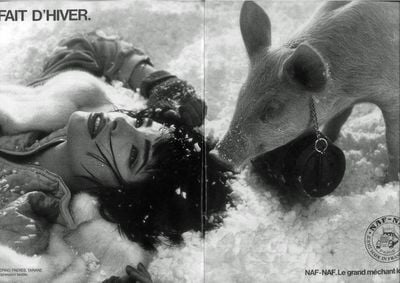
It’s plain to the eye the work is derivative.
And in fact, that was the whole point: Koons brought to three dimensions some of banality of everyday kitsch. In a legal battle spanning four years, Koons’ lawyers argued unsuccessfully that such derivative work was still unique, on several grounds. For instance, he had turned it into three dimensions, added a penguin and goggles on the woman, applied color, changed her jacket, the material representing snow, changed the scale, and much more.
While derivative, with all these new attributes, was the work not then brand new? The French court said non. Koons was found guilty in 2018. And it’s not the first time he was found guilty — in five lawsuits which sprang from this Banality collection, Koons lost three, and another settled out of court.
Unlike other “derivative works” lawsuits of the past, generative models in AI are relying not upon one work of a given artist, but an entire body of millions of images from hundreds of thousands of creators. Photographs are often lumped in with artistic sketches, oil paintings, graphic novel art and more to fashion new styles.
And, while it’s possible to look into the latent layers of AI models and see vectors of numbers, it’s impossible to translate that into something akin to “this new image is 2% based on image A64929, and 1.3% based on image B3929, etc.” An AI model learns patterns from enormous datasets, and those patterns are not well articulated.
Potential Approaches
It would be possible, it seems to me, to pass laws requiring that AI generative models use properly licensed (i.e., copyright-free or royalty-paid images), and then divvy up that royalty amongst its creators. Each artist has a different value for their work, so presumably they’d set the prices and AI model trainers would either pay for those or not.
Compliance with this is another matter entirely; perhaps certification technologies would offer valid tokens once verifying ownership. Similar to the blockchain concept, perhaps all images would have to be traceable to some payment or royalty agreement license. Or perhaps the new technique of Non Fungible Tokens (NFTs) can be used to license out ownership for ingestion during the training phase. Obviously this would have to be scalable, so it suggests automation and a de-facto standard must emerge.
Or will we see new kinds of art comparison or “plagiarism” tools, letting artists compare similarity and influence between generated works and their own creation? Perhaps if a generated piece of art is found to be more than 95% similar (or some such threshold) to an existing work, it will not retain copyright and/or require licensing of the underlying work. It’s possible to build such comparative tools today.
In the meantime, it’s the Wild West of sorts. As has often happened in the past, technology’s rapid pace of advancement has gotten ahead of where legislation and currency and monetary flow is.
What’s Ahead
If you’ve come with me on this journey into AI-generated art in 2022, or have seen these tools up close, you’re like the viewer who’s seen the world wide web in 1994. You’re on the early end of a major wave. This is a revolution in its nascent stage. We don’t know all that’s ahead, and the incredible capabilities of these tools are known only to a tiny fraction of society at the moment. It is hard to predict all the ramifications.
But if prior disintermediation moments are any guide, I’d expect change to happen along a few axes.
First, advancement and adoption will spread from horizontal tools to many more specialized verticals. Right now, there’s great advantage to being a skilled “image prompter.” I suspect that like photography, which initially required extreme expertise to create even passing mundane results, the engines will get better at delivering remarkable images the first pass through. Time an again in technology, generalized “horizontal” applications have concentrated into an oligopoly market of a few tools (e.g., spreadsheets), yet also launched a thousand flowers in much more specialized “vertical” ones (accounting systems, vertical applications, etc.) I expect the same pattern here. These tools have come of age, but only a tiny fraction of people know about them. We’re still in the generalist period. Meanwhile, they’re getting better and better. Right now, these horizontal applications stun with their potential, and are applicable to a wide variety of uses. But I’d expect thousands of specialty, domain specific applications and brand-names to emerge (e.g., book illustration, logo design, storyboard design, etc.) One set of players might generate sketches for creating book covers. Another for graphic novels. Another might specialize in video streaming backgrounds. Not only will this make the training datasets much more specific and outputs even more relevant, but it will allow the brand-names of each engine to better penetrate a specific customer base and respond to their needs. Applications will emerge for specific domains (e.g., automated graphic design for, say, blog posts.)
Second, many artists will demand compensation and seek to restrict rights to their work. Perhaps new guilds will emerge. A new technology and payments system might likely emerge to allow this to scale. Content generally has many ancillary rights, and one of those rights will likely be “ingestion rights” or “training model rights.” I would expect micropayment solutions or perhaps some form of blockchain-based technology to allow photographers, illustrators and artists to protect their work from being ingested into models without compensation. This might emerge as some kind of paywall approach to individual imagery. As is happening in the world of music, the most powerful and influential creative artists may initiate this trend, by cordoning off their entire collective body of work. For instance, the Ansel Adams estate might decide to disallow all ingestion into training models; right now however it’s very difficult to prove whether or not those images were used in the training of any datasets.
Third, regulation might be necessary to protect vital creative ecosystems. If an AI generative machine is able to create works auctioned at Christie’s for $5 million, and it may well soon, what does this do to the ecosystem of creators? It is likely necessary for regulators to protect the ecosystem for creators which feeds derivative engines, restricting AI generative model-makers from just fetching and ingesting any old image.
Fourth, in the near-term, skilled “image prompters” are like skilled photographers, web graphic designers, or painters. Today, there is a noticeable difference between those who know how to get the most of these new tools from those who do not. For the short term, this is likely to “gatekeep” this technology and validate the expertise of designers. I do not expect to this to be extremely durable, however; the quality of output from very unskilled prompters (e.g., yours truly) now meets or exceeds a lot of royalty-free art that’s out there from the likes of Envato or Shutterstock.
Conclusion
Machines now seem capable of visual creativity. While their output is often stunning, under the covers, they’re just learning patterns from data and semi-randomly assembling results. The shocking advancements since just 2015 suggest much more change is on the way: human realism, more styles, video, music, dialogue… we are likely to see these engines pass the artistic “Turing test” across more dimensions and domains.
For now, you need to be plugged into geeky circles of Reddit and Discord to try them out. And skill in crafting just the right prompts separates talented jockeys from the pack. But it’s likely that the power will fan out to the masses, with engines of wow built directly into several consumer end-user products and apps over the next three to five years.
We’re in a new era, where it costs pennies to envision dozens of new images visualizing anything from text. Expect some landmark lawsuits to arrive soon on what is and is not derivative work, and whether such machine-learning output can even be copyrighted. For now, especially if you’re in a creative field, it’s good advice to get acquainted with these new tools, because they’re here to stay.